According to recent research from Cornell, Xbox, and Microsoft Research, while gamers from around the world may have different ideas, this diversity of thinking leads to stronger algorithms that assist audiences globally choose the ideal games.
Researchers demonstrate, with the help of over 5,000 gamers, that predictive models fed on enormous datasets labeled by gamers from diverse nations provide more personalized gaming suggestions than those tagged by gamers from a single country.
Beyond gaming, the team’s findings and related guidelines have extensive utility for researchers and practitioners seeking more internationally applicable data labeling and, as a result, more accurate predictive artificial intelligence (AI) models.
RELATED: Fortnite Users Can Now Qualify For A Share Of The Company’s $245 Million FTC Settlement
“We show that, in fact, you can do just as well, if not better, by diversifying the underlying data that goes into predictive models,” Allison Koenecke, assistant professor of information science at Cornell’s Ann S. Bowers College of Computing and Information Science, said.
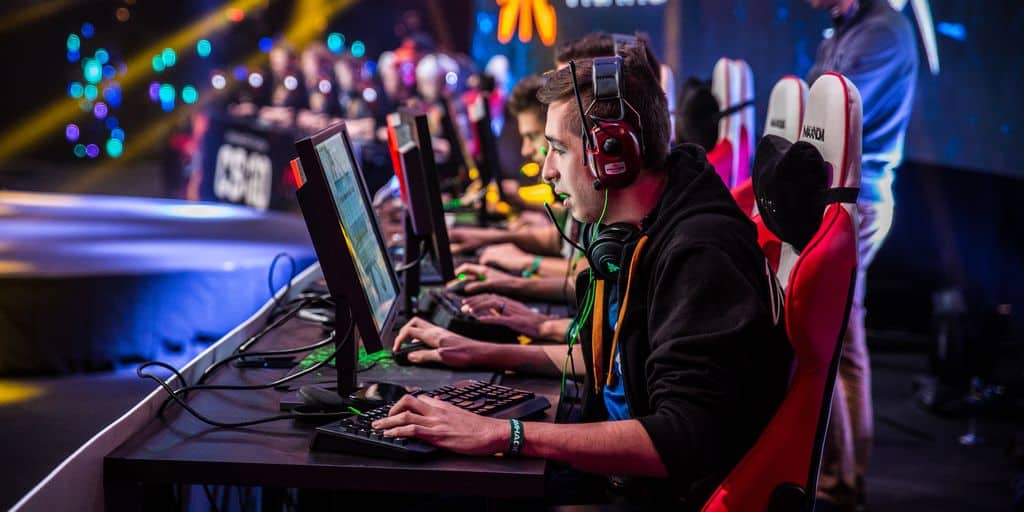
Koenecke is the principal author of the paper “Auditing Cross-Cultural Consistency of Human-Annotated Labels for Recommendation Systems,” which was presented in June at the Association for Computing Machinery Fairness, Accountability, and Transparency (ACM FAccT).
Massive datasets feed into the prediction algorithms that power recommendation systems. The correctness of the model is dependent on the underlying data, particularly the proper labeling of each individual piece inside that vast trove. Crowdsourced workers are increasingly being used by researchers and practitioners to accomplish this labeling for them, however crowdsourced workforces tend to be homogeneous.
RELATED: A Gaming PC With A Beer Fridge Integrated In It Has Been Created By Heineken
Cultural bias can slip in during the data-labeling process, skewing a predictive model designed to serve global audiences, according to Koenecke.
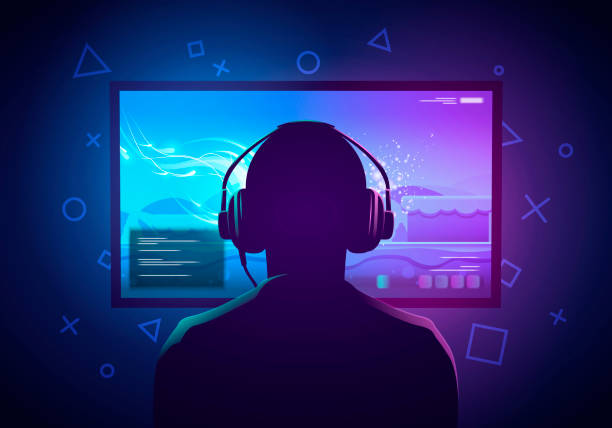
“For the datasets used in algorithmic processes, someone still has to come up with either some rules or just some general idea of what it means for a data point to be labeled in some way,” Koenecke stated. “That’s where this human aspect comes in, because humans do have to be the decision makers at some point in this process.”
To assist label gaming titles, the team polled 5,174 Xbox gamers from around the world. They were asked to assign labels such as “cozy,” “fantasy,” or “pacifist” to games they had played, as well as to examine various characteristics such as whether a title is low or high in complexity, or the difficulty of the game controls.
RELATED: The New PlayStation Console Will Be Available ‘Later This Year’
Some game labels, such as “zen,” which is used to define serene, calming games, were consistently applied across countries; others, such as whether a game is “replayable,” were inconsistently applied. The scientists employed computational approaches to discover that cultural differences among gamers, as well as translational and linguistic oddities of specific labels, contributed to labeling disparities across countries.
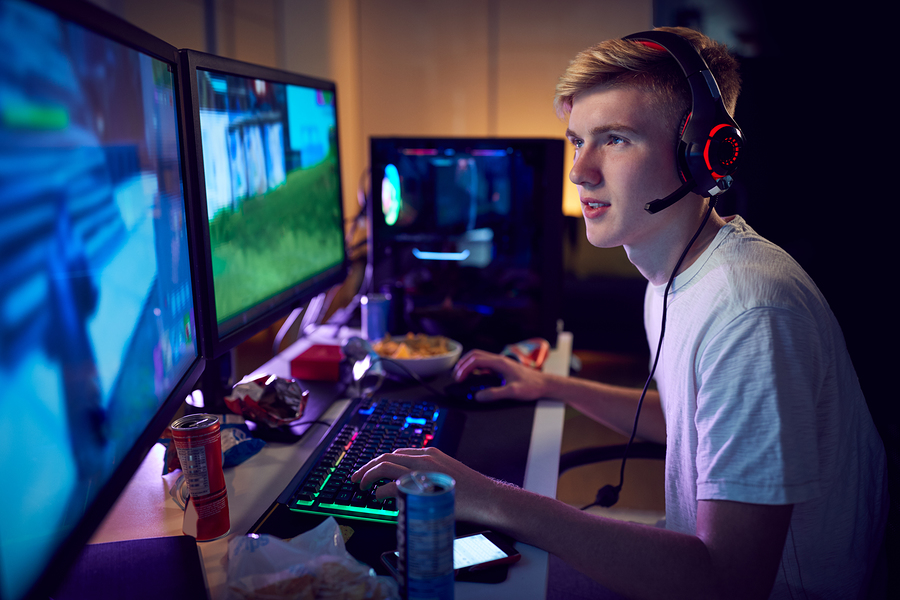
The researchers then created two models that could predict how gamers in each country would identify a specific game—one was fed survey data from globally representative gamers, and the other was fed survey data from solely US gamers. They discovered that the model trained on labels from varied global populations outperformed the other model trained on labels from only American gamers by 8% for gamers worldwide.
“We see improvement for everyone—even for gamers from the U.S.—when the training data is shifted from being entirely U.S.-centric to being more globally representative,” Koenecke stated.
In addition to their findings, the researchers created a framework to help other researchers and practitioners examine underlying data labels for global inclusivity.
“Companies tend to use homogeneous data labelers to do their data labeling, and if you’re trying to build a global product, you’ll run into issues,” Koenecke stated. “With our framework, any academic researcher or practitioner could audit their own underlying data to see if they might be running into issues of representation via their data labels or choices.”
Download The Radiant App To Start Watching!
Web: Watch Now
LGTV™: Download
ROKU™: Download
XBox™: Download
Samsung TV™: Download
Amazon Fire TV™: Download
Android TV™: Download