A new study investigates how artificial intelligence might not only better forecast but also meaningfully expand fresh scientific discoveries.
The researchers created models that can predict human conclusions and the scientists who will make them, according to their findings published in Nature Human Behaviour.
The authors also developed algorithms that avoided human inference in order to generate scientifically promising “alien” theories that would not be examined until the far future, if at all. They contend that the two demonstrations—the first allowing for the acceleration of human discovery and the second identifying and overcoming its blind spots—mean that a human-aware AI would enable moving beyond the current scientific horizon.
“If you build in awareness to what people are doing, you can improve prediction and leapfrog them to accelerate science,” says co-author and Knowledge Lab director James A. Evans. “However, you can also determine what people are unable to do now or will be unable to do for decades or more in the future.” You can help them by supplying them with supplementary intelligence.”
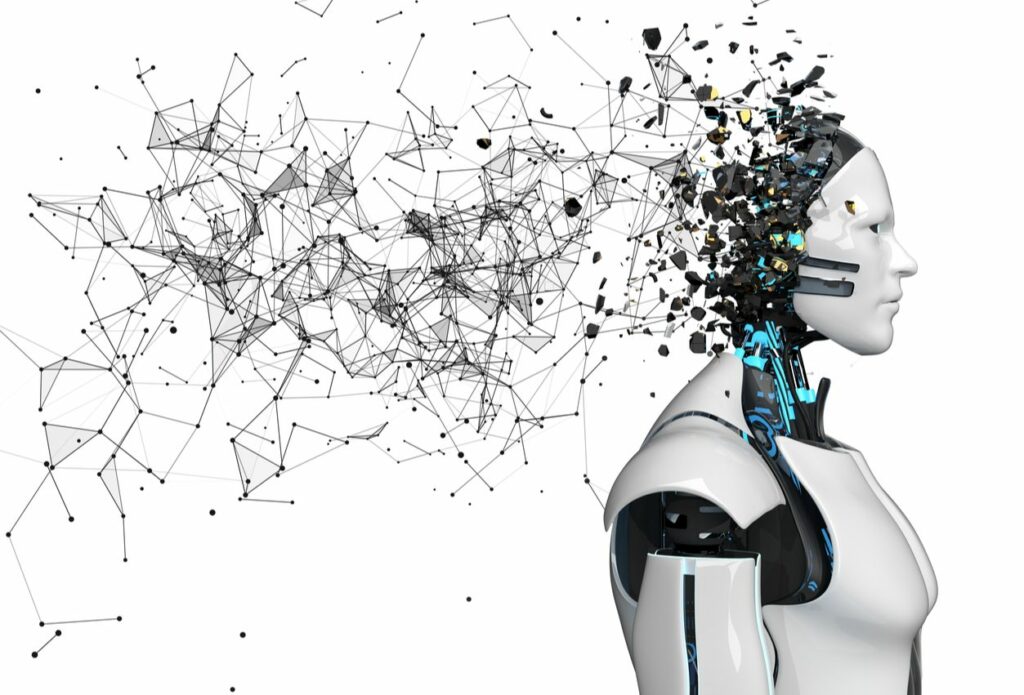
AI models trained on published scientific findings have been used to create valuable materials and targeted cures, but they frequently neglect the distribution of human scientists engaged. The researchers reflected on how humans have competed and collaborated on research throughout history, and they wondered what could be learned if AI programs were explicitly made aware of human expertise: Could we do a better job of complementing collective human capacity by pursuing and exploring places humans haven’t explored?
To answer the challenge, the researchers first built random walks through academic material to replicate reasoning processes. They started with a property, like COVID vaccine, and then moved on to a paper having that same property, another article by the same author, or a material cited in that study.
They ran millions of these random walks, and their approach improved forecasts of future discoveries by 400% over those based just on study content, even when relevant literature was scarce. They could also anticipate the actual persons who would make each of those discoveries with higher than 40% accuracy, because the software knew that the projected human was one of just a few whose experience or relationships linked the property and material in question.
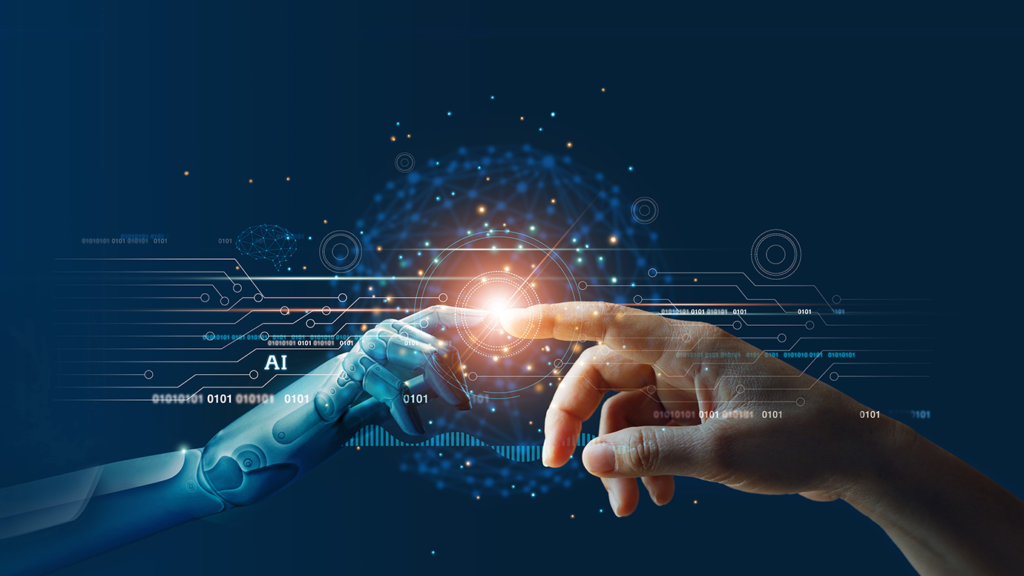
Evans describes the model as a “digital double” of the scientific system, allowing modeling of what is likely to happen and experimentation with different scenarios. He shows how this highlights how scientists adhere to the methods, properties, and persons with which they are familiar.
“It also allows us to learn things about that system and its limits,” he explains. “For example, it suggests that some aspects of our current scientific system, such as graduate education, are not tuned for discovery on average.” They’re programmed to give them a name that will help them acquire a job—to populate the labor market. They do not optimize the discovery of new, technologically significant items. To do so, each pupil would be an experiment, crossing new gaps in the expert landscape.”
In the paper’s second example, they asked the AI model to find predictions that are scientifically plausible but unlikely to be discovered by humans, rather than predictions that are scientifically plausible but unlikely to be discovered by humans.
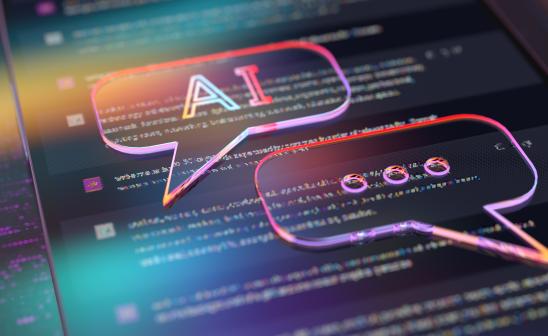
The researchers classified them as alien or complimentary conclusions, which had three characteristics: They are rarely discovered by humans; if they are discovered, it will be many years later when scientific systems reorganize themselves; and alien inferences are, on average, better than human inferences, most likely because humans will focus on squeezing every ounce of discovery from an existing theory or approach before exploring a new one. These models explore wholly new territory since they ignore links and arrangements of human scientific endeavor.
Intelligence has been significantly enhanced.
Evans shows why viewing AI as an attempt to replicate human capacity—based on Alan Turing’s theory of the imitation game, in which people serve as the benchmark of intelligence—doesn’t help scientists speed their ability to solve issues. He believes that a radical enhancement of our collective intelligence, rather than artificial reproduction, is far more likely to help us.
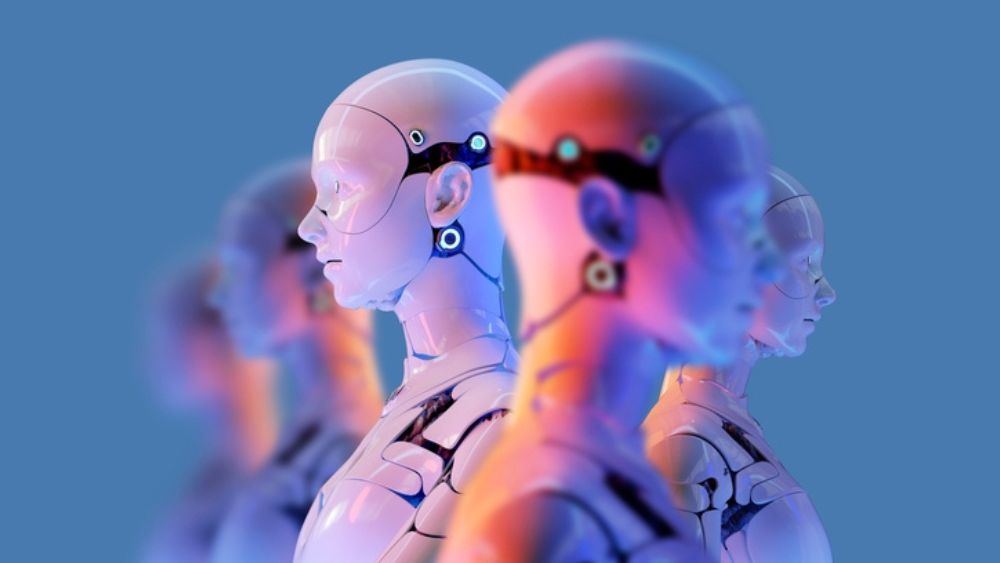
“People in these domains—science, technology, culture—are trying to keep up,” Evans added. “You survive by wielding power over others who use your ideas or technology.” And you get the most out of it by keeping close to the pack. Our models compensate for this bias by developing algorithms that follow signals of scientific plausibility while avoiding the pack.”
Rather than reflecting what human scientists are likely to believe in the near future, using AI to move beyond existing methodologies and collaborations expands human potential and encourages improved research.
“It’s about shifting the AI framing from artificial intelligence to radically augmented intelligence, which necessitates learning more, not less, about individual and collective cognitive capacity,” Evans explained. “When we know more about human understanding, we can explicitly design systems that compensate for its limitations and lead to us collectively knowing more.”
Download The Radiant App To Start Watching!
Web: Watch Now
LGTV™: Download
ROKU™: Download
XBox™: Download
Samsung TV™: Download
Amazon Fire TV™: Download
Android TV™: Download