Researchers at the University of California San Diego School of Medicine have created a method for finding high-affinity antibody medicines that is based on artificial intelligence (AI).
Researchers utilized the method in the study, which was released on January 28, 2023 in Nature Communications, to discover a novel antibody that binds a significant cancer target 17-fold more tightly than an antibody medication currently on the market. According to the scientists, the pipeline may hasten the development of new medications to treat conditions like COVID-19 and rheumatoid arthritis as well as cancer.
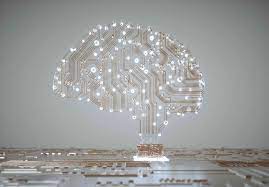
A successful drug requires an antibody to bind firmly to its target. Researchers usually start with a known antibody amino acid sequence in order to locate these antibodies, and then they use bacterial or yeast cells to make a series of novel antibodies with variations on that sequence. Then, the mutants’ capacity to bind the target antigen is assessed. The best-performing subset of antibodies is then put through another round of modifications and assessments, and this cycle is repeated until a short list of tightly-binding finalists is established.
Many of the produced antibodies still show poor results in clinical trials despite this time-consuming and expensive process. To expedite and streamline these efforts, UC San Diego researchers created a cutting-edge machine learning algorithm for the current study.
Similar to other approaches, this one begins with creating an initial library of roughly 500,000 potential antibody sequences and screening them for their affinity to a particular protein target. The dataset is fed into a Bayesian neural network, which may interpret the data and utilize it to forecast the binding affinity of new sequences, rather than repeatedly repeating this procedure.
professor of Cellular and Molecular Medicine at UC San Diego School of Medicine.
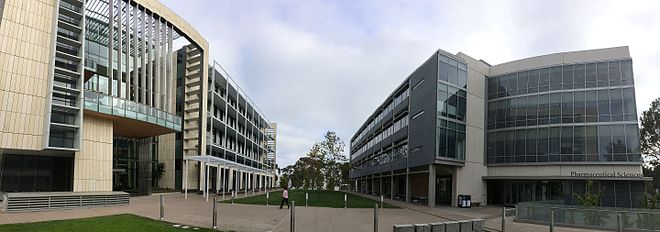
One particular advantage of their AI model is its ability to report the certainty of each prediction. “Unlike a lot of AI methods, our model can actually tell us how confident it is in each of its predictions, which helps us rank the antibodies and decide which ones to prioritize in drug development,” said Wang.
To validate the pipeline, project scientists and co-first authors of the study Jonathan Parkinson, PhD, and Ryan Hard, PhD, set out to design an antibody against programmed death ligand 1 (PD-L1), a protein highly expressed in cancer and the target of several commercially available anti-cancer drugs. Using this approach, they identified a novel antibody that bound to PD-L1 17 times better than atezolizumab (brand name Tecentriq), the wild-type antibody approved for clinical use by the U.S. Food and Drug Administration.
The researchers are now using this approach to identify promising antibodies against other antigens, such as SARS-CoV-2. They are also developing additional AI models that analyze amino acid sequences for other antibody properties important for clinical trial success, such as stability, solubility and selectivity.
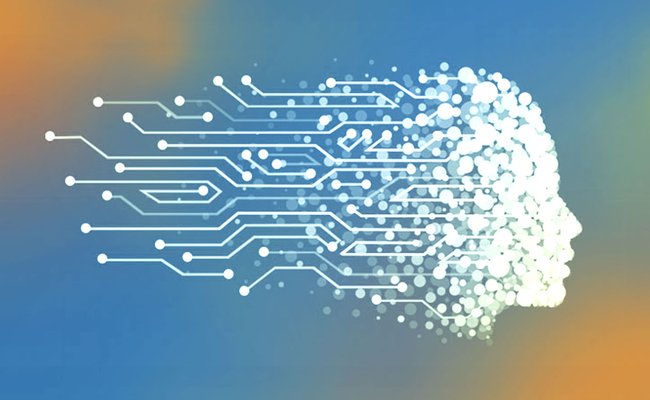
“By combining these AI tools, scientists may be able to perform an increasing share of their antibody discovery efforts on a computer instead of at the bench, potentially leading to a faster and less failure-prone discovery process,” said Wang. “There are so many applications to this pipeline, and these findings are really just the beginning.”
Funding for this research came, in part, from the National Institutes of Health (grants R01GM111941 and R21AI158114).
Disclosures: All three authors are co-authors on USPTO provisional patent applications 63432836 and 63431556, which cover the tight-binding antibody sequences described herein and the pipeline, filed with the assistance of the University of California San Diego.
“By combining these AI tools, scientists may be able to perform an increasing share of their antibody discovery efforts on a computer instead of at the bench, potentially leading to a faster and less failure-prone discovery process.” Wei Wang, PhD, professor of cellular and molecular medicine at UC San Diego School of Medicine.
“By combining these AI tools, scientists may be able to perform an increasing share of their antibody discovery efforts on a computer instead of at the bench, potentially leading to a faster and less failure-prone discovery process.” Wei Wang, PhD, professor of cellular and molecular medicine at UC San Diego School of Medicine.
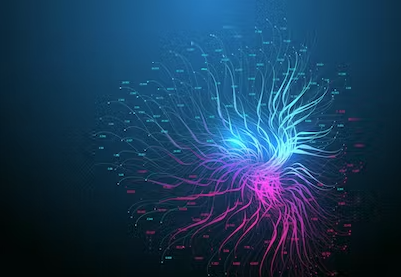
According to senior author Wei Wang, PhD, professor of Cellular and Molecular Medicine at UC San Diego School of Medicine, “using our machine learning methods, these successive rounds of sequence mutation and selection may be carried out swiftly and efficiently on a computer instead of in the lab.”
Their AI model’s capacity to report the accuracy of each forecast is one of its key advantages. We can rank the antibodies and choose which ones to prioritize in medication development since, unlike many AI techniques, our model can truly communicate how confident it is in each of its predictions, according to Wang.
Jonathan Parkinson, PhD, and Ryan Hard, PhD, project scientists and co-first authors of the study, set out to develop an antibody against programmed death ligand 1 (PD-L1), a protein that is heavily expressed in cancer and the target of a number of commercially available anti-cancer drugs, in order to validate the pipeline. By employing this method, scientists discovered a new antibody that bound to PD-L1 17 times more effectively than atezolizumab (Tecentriq), the wild-type antibody that the U.S. Food and Drug Administration has licensed for use in clinical trials.
This method is currently being used by the researchers to find potential antibodies against additional antigens, such as SARS-CoV-2. They are also creating new AI models that examine amino acid sequences for other antibody qualities including stability, solubility, and selectivity that are crucial for the outcome of clinical trials.
By combining these AI techniques, Wang stated, “scientists may be able to execute a greater proportion of their antibody discovery work on a computer instead of at the bench, perhaps leading to a faster and less prone to failure discovery process.” “This pipeline has a plethora of applications, and these results are truly only the tip of the iceberg.”
Download The Radiant App To Start Watching!
Web: Watch Now
LGTV™: Download
ROKU™: Download
XBox™: Download
Samsung TV™: Download
Amazon Fire TV™: Download
Android TV™: Download